FREE Labs Fishbone Phone Background
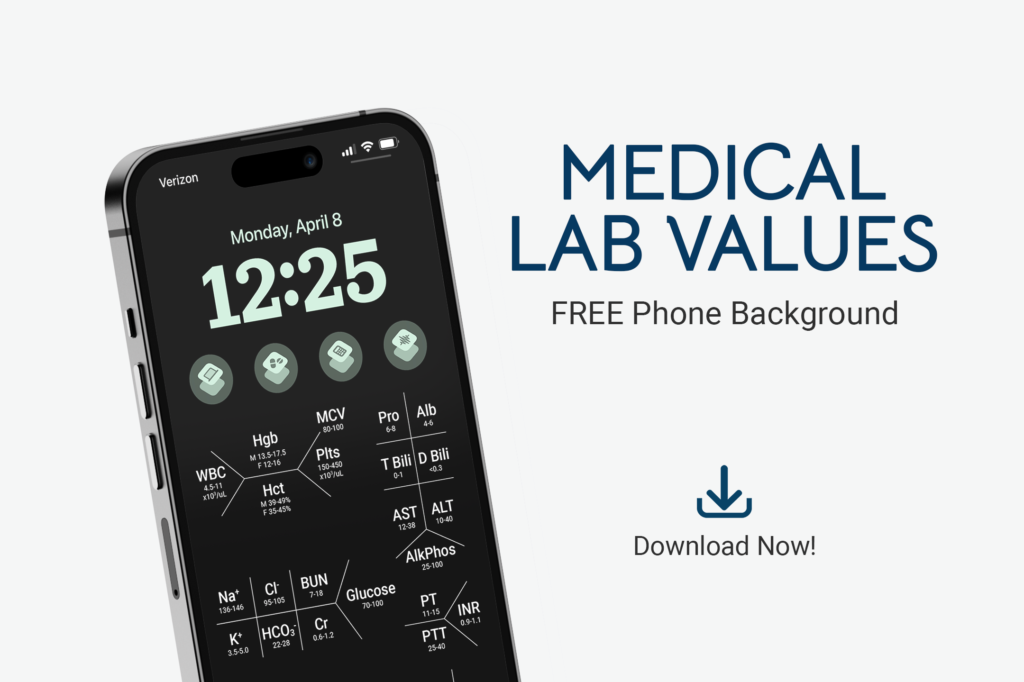
The “fishbone” or “skeleton” diagram is essential for medical professionals who want to rapidly organize laboratory test results. Download the FREE phone background now!
Personalized Medicine through Machine Learning: Tailoring Treatments for Individuals

In the pursuit of improved patient outcomes, personalized medicine through machine learning emerges as a promising avenue. By leveraging predictive analytics and individualized data, healthcare professionals can optimize interventions, leading to more favorable results for patients.
Understanding C Scores in AI Medical Calculators

In the realm of medical clinical assessment tools, machine learning has become an invaluable asset for healthcare professionals. One key metric often used to evaluate the performance of these tools is the C score. Here, we will delve into the intricacies of interpreting C scores and understanding their significance in the context of medical applications. What is a C Score? The C score, also known as the area under the receiver operating characteristic curve (AUC-ROC), is a common metric used to assess the performance of a machine learning model. It is particularly relevant in medical applications where the accuracy of predictions is crucial. The AUC-ROC curve is a graphical representation of a model’s ability to discriminate between positive and negative cases across different threshold values. How do you interpret C Score? The curve represents the trade-off between true positive rate (sensitivity) and false positive rate (1-specificity) at various thresholds. A higher AUC indicates better discrimination ability. An AUC of 1.0 implies perfect discrimination, while 0.5 suggests performance equivalent to random chance. While a high AUC is desirable, it doesn’t provide a complete picture of a model’s performance. It’s essential to consider other metrics such as precision, recall, and accuracy to evaluate the overall effectiveness of the model. The interpretation of C scores depends on the context of the medical application. For instance, in cancer diagnosis, a high sensitivity might be more critical than specificity. Consider the clinical implications of false positives and false negatives. Some medical scenarios may prioritize minimizing one over the other. Does a higher C score mean one calculator is better than another? No, higher C Score does not equal higher accuracy or a better calculator. Compare the C scores of different models to identify the most suitable one for a specific clinical application. Conduct thorough validation and cross-validation to ensure robustness and generalizability. Be aware of potential pitfalls such as imbalanced datasets, overfitting, and the impact of skewed class distribution on C scores. Interpreting C scores in a medical clinical assessment tool is a nuanced process that requires a comprehensive understanding of the AUC-ROC curve and its implications. Healthcare professionals must approach these scores with a critical mindset, considering the specific context of the medical application and other relevant metrics. By doing so, they can harness the power of machine learning to enhance clinical decision-making and patient outcomes.
Beyond Numbers: How Machine Learning is Redefining Medical Calculators
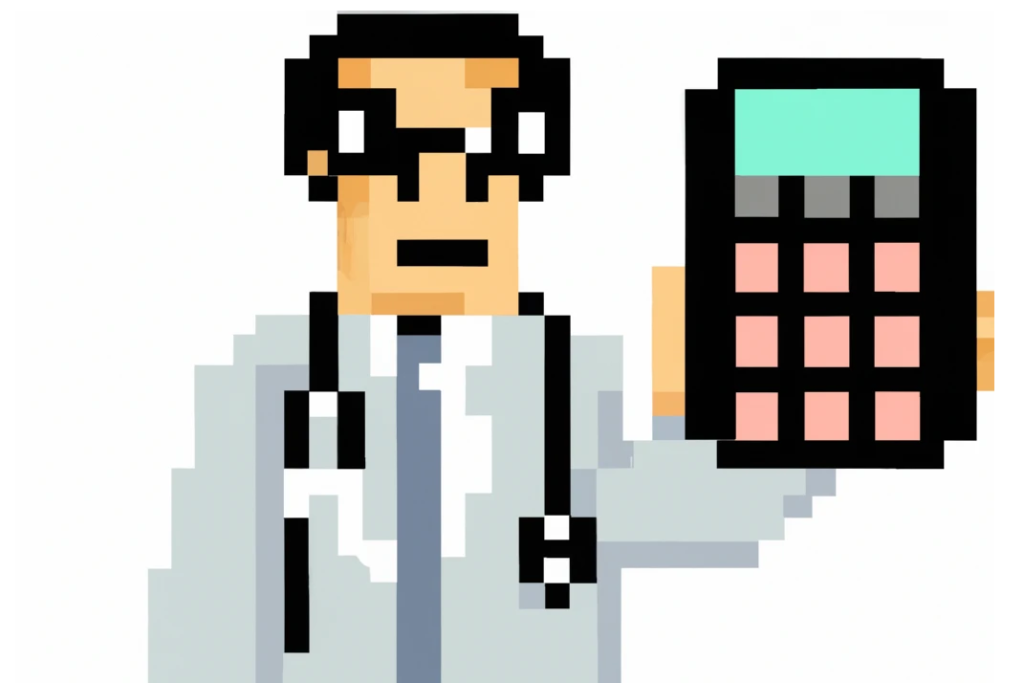
In an era where technology and healthcare are becoming increasingly intertwined, the advent of machine learning (ML) in medical tools marks a revolutionary stride forward. Particularly, machine learning-based medical calculators have emerged as pivotal assets in the healthcare sector, offering a blend of innovative computation and clinical expertise. These calculators are more than just digital tools; they are intelligent systems designed to assist in diagnostic and therapeutic decision-making processes.